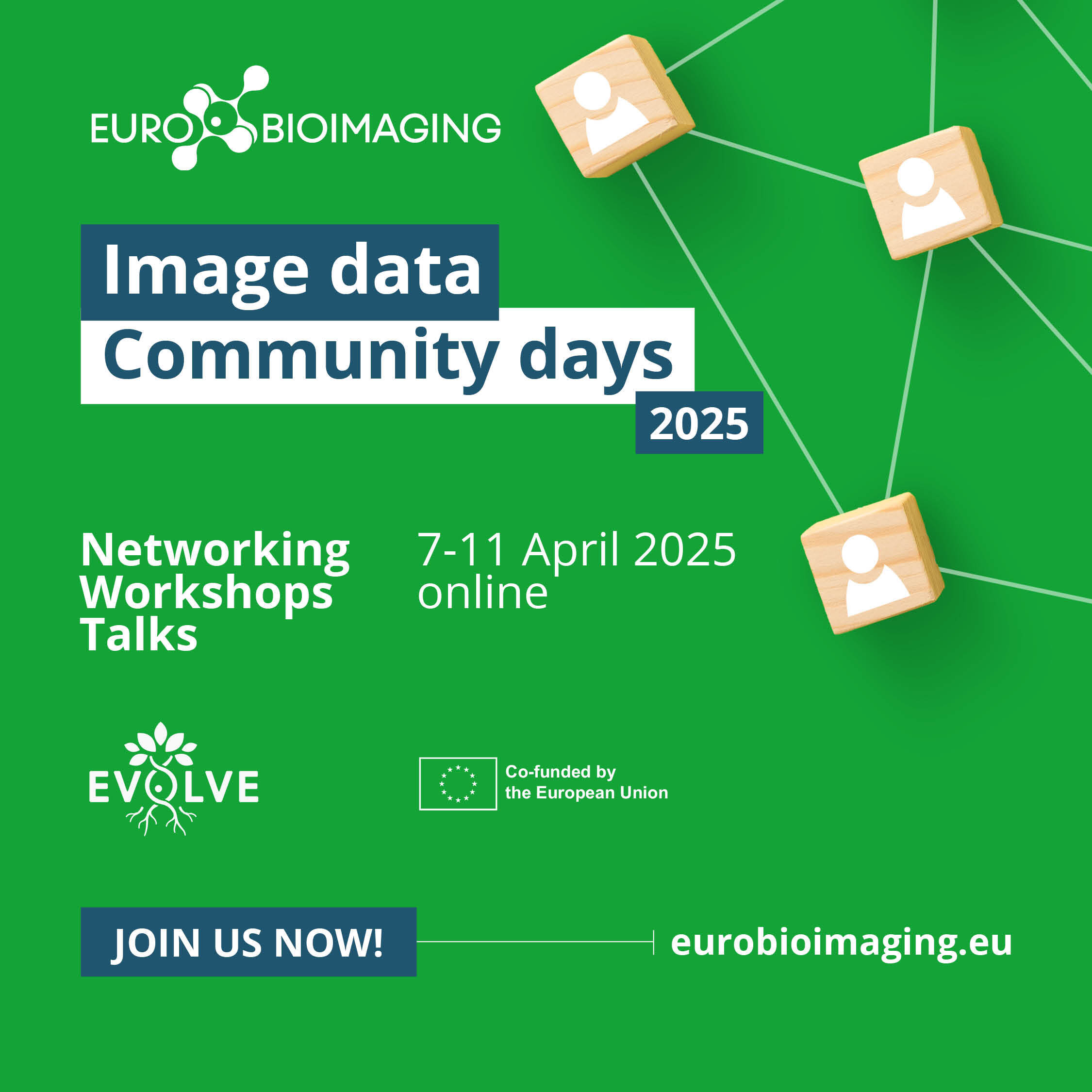
Harnessing Differentiable Data Models for Machine Learning Integration in Microscopy
Imaging technologies are becoming increasingly complex and ever more expensive, reducing the general accessibility and potential reach of cutting-edge techniques. The Special Edition Virtual Pub “Open Hardware in Imaging,” in collaboration with the Euro-BioImaging Industry Board, will highlight developments from scientists and companies who are committed to making biological & biomedical imaging hardware and software solutions openly available to a wide audience.
When: September 22, 2023, from 13:00-15:00 CEST
Where: Online
At this event, Luis Oala, Dotphoton, will present Harnessing Differentiable Data Models for Machine Learning Integration in Microscopy - (full abstract below). Hear this talk and others like it on September 22!
ABSTRACT
Harnessing Differentiable Data Models for Machine Learning Integration in Microscopy
Luis Oala
Dotphoton
The integration of machine learning with data acquisition in microscopy has emerged as a promising frontier. This abstract summarizes key concepts, results, and implications from a study exploring the potential of physically accurate, differentiable data models in enhancing image acquisition and model training.
Our study introduces drift synthesis, forensics, and optimization, all underpinned by a differentiable data model. Drift synthesis creates physically faithful test cases for model selection. Forensics identifies areas for performance improvement. Optimization employs machine learning to optimize image processing by backpropagating from the task model through the ISP to the raw sensor data.
The microscopy experiments demonstrate the effectiveness of these concepts. Task models trained on physically faithful data models outperformed those trained on common corruptions. Changes in the black level configuration and denoising parameters pose the greatest risk for task model performance. The drift optimization experiment demonstrated how raw data and a differentiable data model can be used to identify unfavorable data models that should be avoided during task model deployment.
These findings suggest that machine-optimized processing could lower hardware costs, making high-quality ISPs more affordable. The use of physically accurate data models for dataset drift validation underscores the importance of precise data models in maintaining task model performance.
In the context of the Open Hardware in Imaging workshop, these findings highlight the potential of machine learning and physically accurate, differentiable data models to innovate image acquisition and model training in microscopy. The differentiable nature of the data model allows for the integration of classical machine learning with data acquisition, opening up new possibilities for research and application in microscopy.
For more details, refer to the full paper Oala, Luis, Marco Aversa, Gabriel Nobis, Kurt Willis, Yoan Neuenschwander, Michèle Buck, Christian Matek et al. "Data Models for Dataset Drift Controls in Machine Learning With Optical Images." Transactions on Machine Learning Research (2023).
More news from Euro-BioImaging
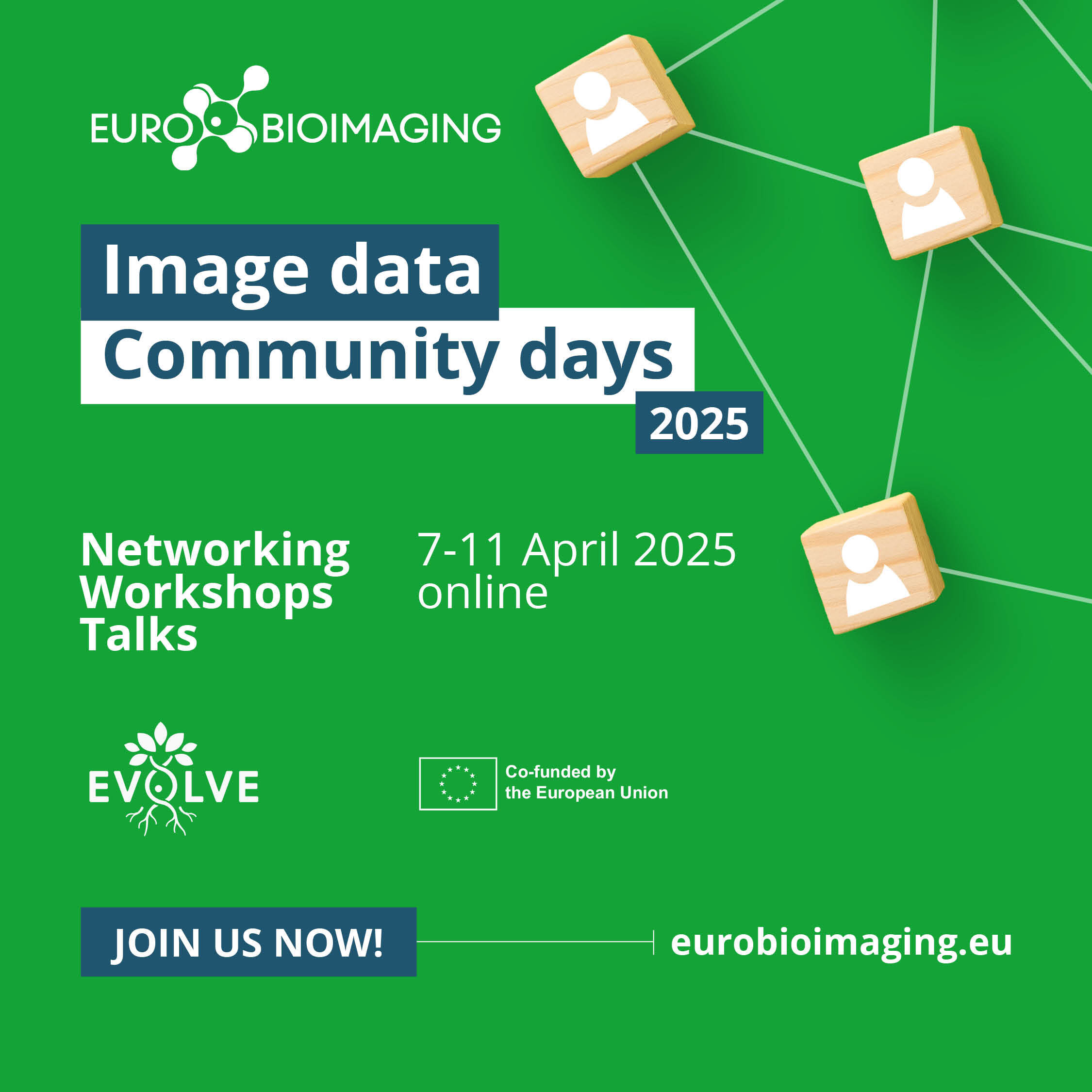
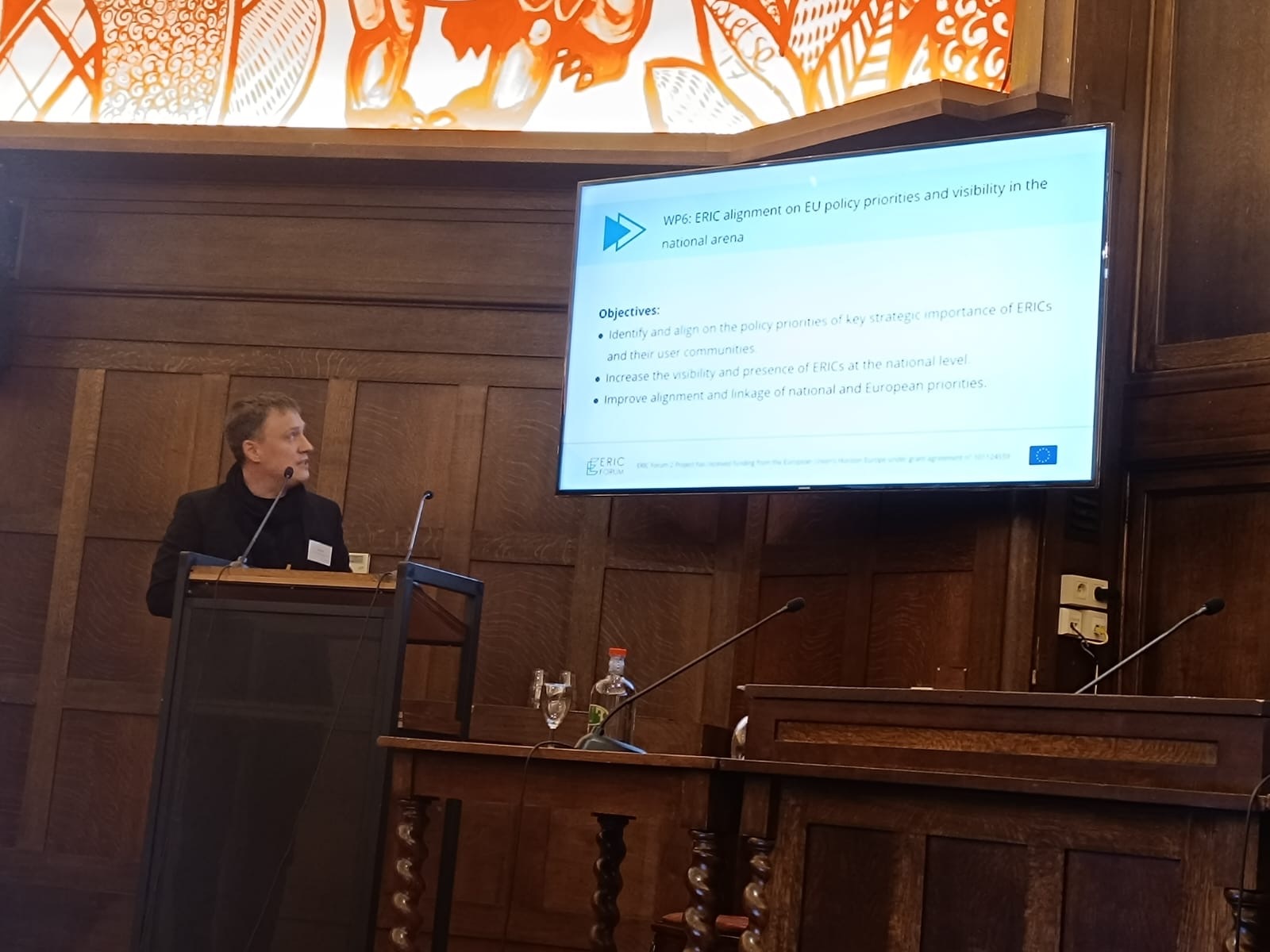
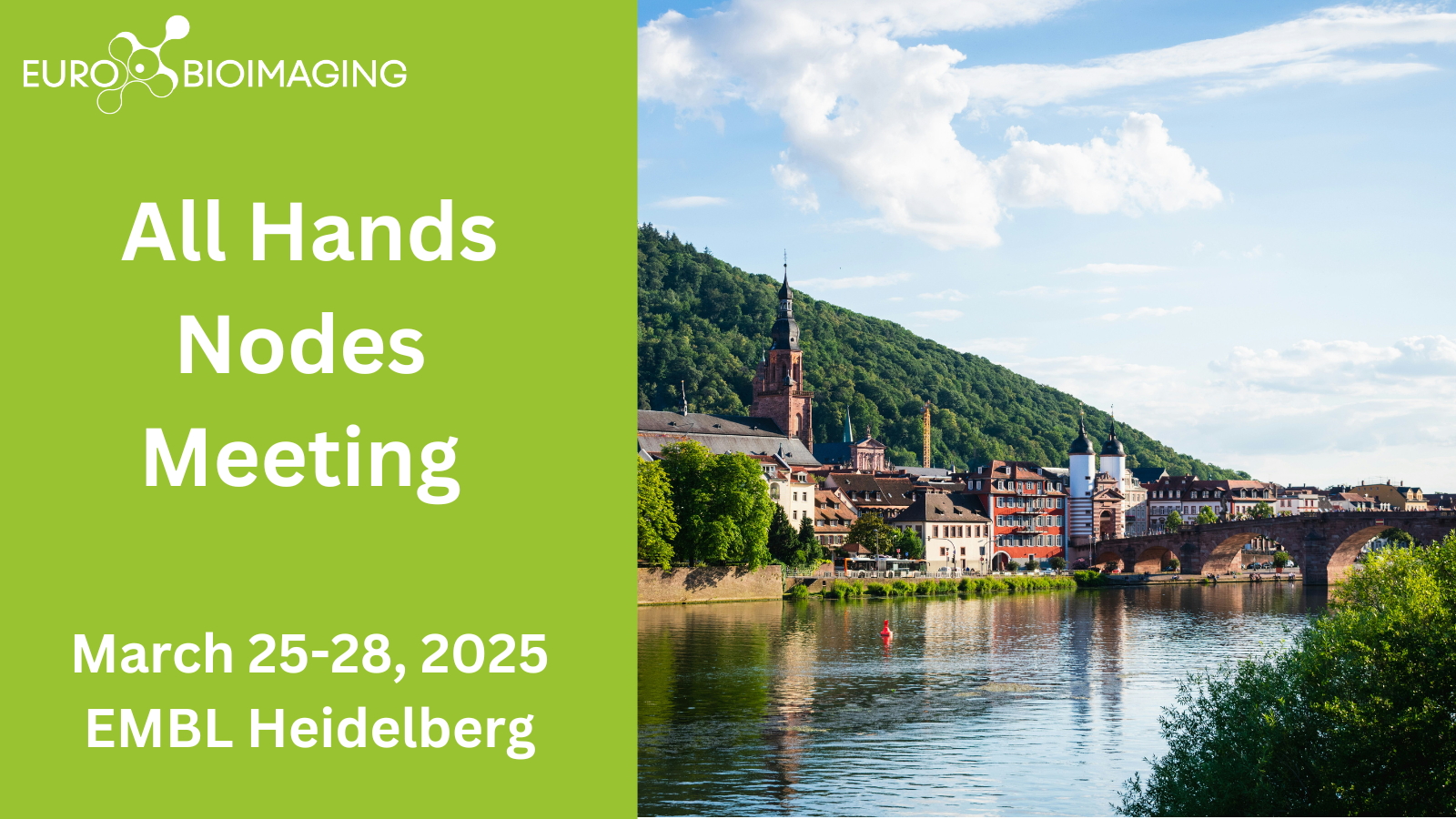